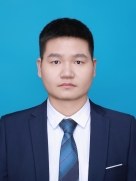 |
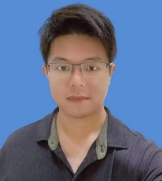 |
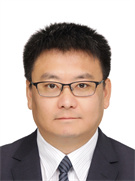 |
Assoc. Prof. Fengchao Xiong 熊凤超副教授 Nanjing
University of Science and Technology, China 南京理工大学 |
Assoc. Prof. Jiaxin Cai
蔡加欣副教授 Xiamen University of Technology,
China 厦门理工学院 |
Assoc. Prof. Jianwei Zhang 张建伟副教授 Iwate University, Japan 岩手大学
|
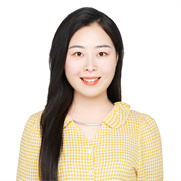 |
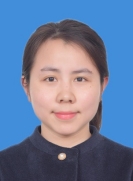 |
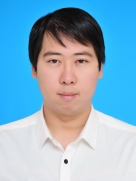 |
Asst. Prof. Wei Tang
唐伟助理教授 Tongji University, China 同济大学
|
Asst. Prof. Huiqi Deng 邓辉琦助理教授 Xi'an Jiaotong
University, China 西安交通大学 |
Assoc. Prof. Hongyuan Jing 景竑元副教授
Beijing Union University 北京联合大学 |
Invited
Speakers of ICCPR 2024 |
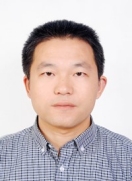 |
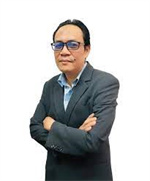 |
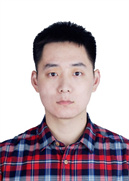 |
Prof. Yangli Jia
贾仰理教授
Liaocheng University, China
聊城大学
|
Assoc. Prof. Mohamad Yusof Darus
Mohamad Yusof Daru副教授
Universiti Teknologi MARA, Malaysia
玛拉工艺大学
|
Assoc. Prof. Bing Cao
曹兵副教授
Tianjin University, China
天津大学
|
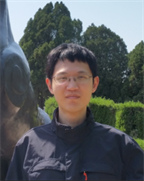 |
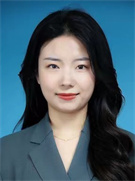 |
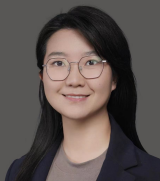 |
Assoc. Prof. Bo Ren
任博副教授
Nankai University, China
南开大学
|
Assoc. Prof. Fan Qi
亓帆副教授
Tianjin University of Technology, China
天津理工大学
|
Assoc. Prof. Yang Gao
高扬副教授
Beijing Institute of Technology, China
北京理工大学
|
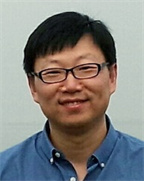 |
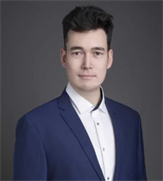 |
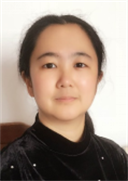 |
Assoc. Prof. Yang Li
李杨副教授
Nanjing University, China
南京大学
|
Asst. Prof. Pascal Lefèvre
Pascal Lefèvre助理教授
Xi’an Jiaotong-Liverpool University, China
西交利物浦大学
|
Prof. Xin Wang
王鑫教授
Tianjin University of Technology, China
天津理工大学
|